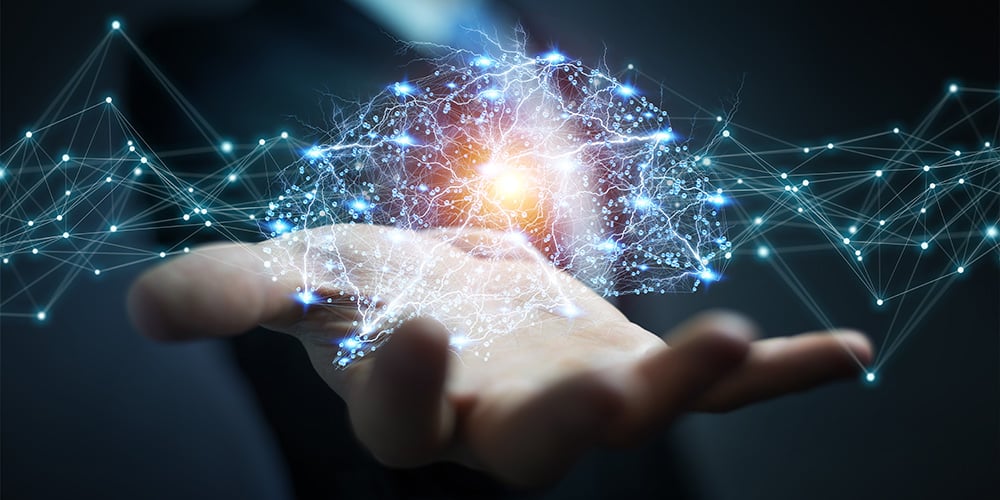
Background
Modelling techniques for bank risk management have always been an important element, with greater emphasis during the last two decades. Well established risk quantification methods are used by banking institutions within their capital calculation, provisioning, forecasting and stress testing, pricing and decision making.
The significant improvement in data processing and computational capabilities has resulted in an increasing industry trend to use more advanced techniques in risk identification and quantification. The trend has been strongest in the area of decision making (non-regulatory) models.
However, recent publications on regulatory models from the EBA (European Banking Authority) and PRA (Prudential Regulation Authority) are developing advanced method use cases. An increased focus on Artificial Intelligence (AI) and machine learning (ML) methods highlights the need for bank risk management to understand the capabilities of advanced modelling techniques.
Within Credit Risk, a role for ML models is becoming more and more relevant across several areas. Examples include:
- Deciding how to categorise or rank order loans or borrowers;
- Identifying the cohort of loans that are most vulnerable;
- Designing efficient credit sanctioning and review processes and
- Identifying emerging risks in a dynamic way.
Having the ability to process data efficiently and help institutions to make better informed decisions in a timely manner represents a few benefits of advanced modelling techniques.
Executive Summary
Our focus in this paper is to develop decision making models using a range of advanced machine learning techniques. We explore three different methodologies to measure the discriminatory power between good and bad borrowers using a credit card portfolio dataset. The main hypothesis is that advanced modelling techniques lead to more efficient estimates and higher discriminatory power.
The first section reviews the applicable regulatory information. Recent regulatory publications and initiatives acknowledge a need to address advanced modelling techniques use cases in bank risk management. A focus on the regulatory capital models shows a relatively conservative regulatory position. The second section provides the definitions on decision making and machine learning principles.
In the third section, three models with varying complexity levels are applied to the credit card dataset. An assessment is performed on the ability to discriminate borrowers repayment status between good (performing) and bad (nonperforming). Model output confirms the hypothesis that applying more advanced ML techniques significantly improves model discriminatory power, leading to more efficient rank ordering. A final section provides a benefit analysis (pros and cons) of using ML techniques in this area.