Sign up for expert insights, industry trends, and key updates—delivered straight to you.
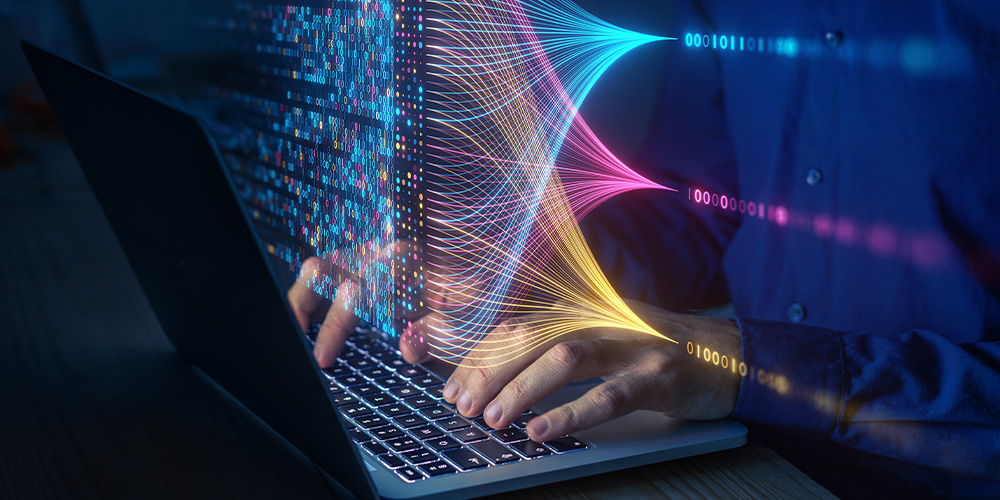
What is AI Literacy?
Artificial intelligence (‘AI’) and machine learning (‘ML’) are transforming the landscape of actuarial science. As these technologies become increasingly integral to the profession, actuaries must develop AI literacy, which involves grasping the key concepts, tools, and applications of AI and ML.
This includes understanding data handling, model building, validation, implementation, and the ethical considerations of AI. With AI literacy, actuaries can leverage these technologies to enhance predictive analytics, automate routine tasks, and improve models for risk assessment, pricing, and forecasting.
Why AI Literacy is Essential for Actuaries
The actuarial profession is rapidly evolving, driven by the need for more accurate and efficient solutions. Staying ahead requires actuaries to harness the power of AI and ML. Actuaries who are proficient in AI can enhance their decision-making processes, collaborate effectively with data scientists, and maintain a competitive edge in the industry.
Developing AI literacy enables actuaries to address complex challenges, drive innovation, and stay responsive to the profession’s changing demands.
This article serves as an introduction to AI literacy for actuaries, setting the stage for further exploration of this topic in subsequent articles.
Core Components of AI Literacy
Developing AI literacy requires actuaries to master key areas that are essential for effectively utilising AI technologies in their work.
1. Foundational Knowledge
A solid foundation in the basic concepts of AI and ML is essential for actuaries. This includes understanding what AI is, how it works, and the types of problems it can solve. Key terminologies such as supervised and unsupervised learning, neural networks, and deep learning should be familiar.
- Supervised Learning: Involves training a model on a labeled dataset, where each data point is paired with a known outcome (label). The model learns to predict the outcome based on the input features. Common applications include classification and regression tasks.
- Unsupervised Learning: Involves training a model on data without labelled outcomes. This is used for clustering and association tasks.
- Neural Networks and Deep Learning: Neural networks are a series of algorithms that attempt to recognize underlying relationships in a set of data through a process that mimics the way the human brain operates. Deep learning, a subset of ML, uses multi-layered neural networks to analyse various factors of data.
2. Data Handling and Preprocessing
Effective AI applications depend on the quality of data. Actuaries need to be proficient in data collection, cleaning, and integration processes to ensure the data used in AI models is accurate and reliable.
- Data Collection: Involves gathering data from various sources, including internal databases, third-party providers, and publicly available datasets. For actuaries, this might include policyholder information, claims data, economic indicators, and other relevant financial data.
- Data Cleaning: The process of fixing or removing incorrect, corrupted, incorrectly formatted, duplicate, or incomplete data within a dataset.
- Data Integration: Combining data from different sources and providing a unified view. This step is crucial for creating comprehensive datasets that AI models can learn from. Integrating data from various sources allows actuaries to have a more holistic view of risks and helps in creating more precise models.
3. Model Building
Understanding various ML techniques is essential for actuaries to build and apply AI models effectively. Key techniques include regression, classification, clustering, and advanced algorithms like decision trees and random forests.
- Regression: A statistical method used in finance, investing, and other disciplines that attempt to determine the strength and character of the relationship between one dependent variable and a series of other variables. Actuaries use regression analysis to model relationships between different risk factors and outcomes, such as predicting claim amounts based on policyholder characteristics.
- Classification: A process related to categorisation, the problem of identifying to which of a set of categories (subpopulations) a new observation belongs. In actuarial work, classification models can be used to categorise policyholders into different risk groups or to identify fraudulent claims.
- Clustering: A task of dividing the population or data points into several groups, such that data points in the same groups are more similar to other data points in the same group than those in other groups. Clustering can help actuaries segment customers or identify patterns in claims data that might not be immediately apparent.
- Advanced Algorithms: Techniques such as decision trees and random forests can handle complex interactions between variables and provide interpretable results. These algorithms can enhance traditional actuarial models by uncovering deeper insights and improving predictive accuracy.
4. Model Validation
Ensuring that AI models perform well on unseen data is critical. Model validation techniques help in assessing the robustness and reliability of the models. Proper validation ensures that the models will perform accurately not just on the training data, but also on new, unseen data.
- Cross-Validation: A technique where the dataset is divided into k subsets, and the model is trained k times, each time using a different subset as the validation set and the remaining (k-1) subsets as the training set. An advantage of this approach is that it helps in detecting overfitting, as the model is tested on multiple different subsets of the data.
- Bootstrapping: A method that involves repeatedly sampling from the data (with replacement) and assessing model performance on these samples. This is useful for estimating the confidence intervals of model predictions.
- Model Monitoring: Once deployed, models need to be continuously monitored to ensure they remain accurate over time and adapt to new data. This ensures the model continues to perform well in a changing environment and helps identify when a model update or retraining is necessary.
5. Model Implementation
Model deployment is a critical phase in the lifecycle of AI models, ensuring they deliver value in real-world applications. For actuaries, this involves moving predictive models from development to production environments where they can be used for tasks such as risk assessment, pricing, and claims processing.
Key steps in the deployment process include preparing the production environment, exporting and versioning models, integrating with existing systems where appropriate, and automating data pipelines. Actuaries must also conduct thorough testing (unit, integration, and acceptance testing) to ensure the model functions correctly under real-world conditions.
Once deployed, ongoing monitoring and maintenance are crucial to ensure models perform reliably and adapt to new data. Actuaries need to implement performance monitoring, data drift detection, and regular retraining to keep models accurate and relevant.
Security measures, such as access control and data encryption, are also vital to protect sensitive information and comply with regulatory requirements. Scalability, reliability, comprehensive documentation, and user feedback loops further enhance the deployment process, ensuring that AI models remain effective and ethically sound in their predictions.
By adhering to these best practices, actuaries can maximise the benefits of AI in their workflows, improving decision-making and operational efficiency.
6. Ethics and Bias in AI
AI applications must be developed and deployed ethically to avoid biases that can lead to unfair or discriminatory outcomes. Actuaries must understand how to ensure fairness, transparency, and accountability in AI models.
- Fairness and Transparency: Ensuring that AI models do not inadvertently discriminate against certain groups and that their decision-making processes are understandable. This involves regularly auditing models for bias and implementing measures to correct any identified issues.
- Regulatory Compliance: Adhering to laws and guidelines that govern the use of AI, especially in sensitive areas like insurance underwriting. This includes complying with data protection regulations, such as the EU AI Act and GDPR, and industry-specific standards.
- Governance and Accountability: Establishing clear lines of responsibility for the development, deployment, and monitoring of AI models. This includes documenting decision-making processes and maintaining transparency with stakeholders.
- Bias Mitigation: Implementing techniques to detect and reduce bias in AI models, such as fairness-aware algorithms, reweighting data, or adjusting model parameters. Regularly updating models and retraining with diverse datasets can also help mitigate bias over time.
By mastering these core components, actuaries can effectively integrate AI into their workflows, enhancing their ability to analyse complex data, automate routine tasks, and develop more accurate predictive models while ensuring ethical considerations are met.
7. Regulatory Landscape for AI in Actuarial Science
As AI becomes increasingly integrated into actuarial practices, it is essential to understand the regulatory landscape governing its use. Regulations are designed to ensure the ethical application of AI, protect individuals' rights, and maintain transparency and accountability in decision-making processes.
For actuaries, staying informed about relevant regulations is crucial to ensure compliance and uphold professional standards.
- EU AI Act: The recent EU AI Act, introduced to create a legal framework for AI applications, is particularly relevant for actuaries operating in Ireland and across Europe. This regulation categorises AI systems based on their risk levels: unacceptable risk, high risk, and limited or minimal risk. Actuarial applications often fall into the high-risk category, especially when they influence decisions in areas like insurance underwriting and claims processing.
- General Data Protection Regulation (GDPR): Although not specific to AI, GDPR plays a crucial role in how AI models handle personal data. Actuaries must ensure that AI systems comply with GDPR principles, such as data minimisation, accuracy, and the right to explanation.
- Irish Data Protection Act 2018: This act further enforces GDPR in Ireland and introduces additional provisions specific to the Irish context such as the requirement for a consent declaration for health data research. Actuaries must be aware of these regulations to ensure full compliance when developing and deploying AI models.
Conclusion
AI literacy is no longer optional for actuaries; it is an essential skill set that enhances traditional actuarial practices and opens new opportunities. By mastering foundational knowledge, data handling, model building, validation, deployment, and understanding ethical implications, actuaries can effectively integrate AI into their workflows.
This article serves as an introduction to the core components of AI literacy for actuaries, setting the stage for further exploration and deeper understanding of each area in subsequent articles. As actuaries continue to develop and apply these skills, they will be better equipped to navigate the future of the actuarial profession and leverage AI to drive innovation and impact.
How Grant Thornton can help
If you need support in any of these areas, please don’t hesitate to reach out:
- Predictive Modelling for Claims and Pricing
- Reserving with Machine Learning
- Anomaly Detection in Claims Data
- Model Optimisation and Comparison
- Automated Data Cleaning and Preprocessing
- Natural Language Processing (NLP) for Document Analysis
- Stochastic Modelling with AI
We’re here to deliver tailored expertise to address your specific challenges.