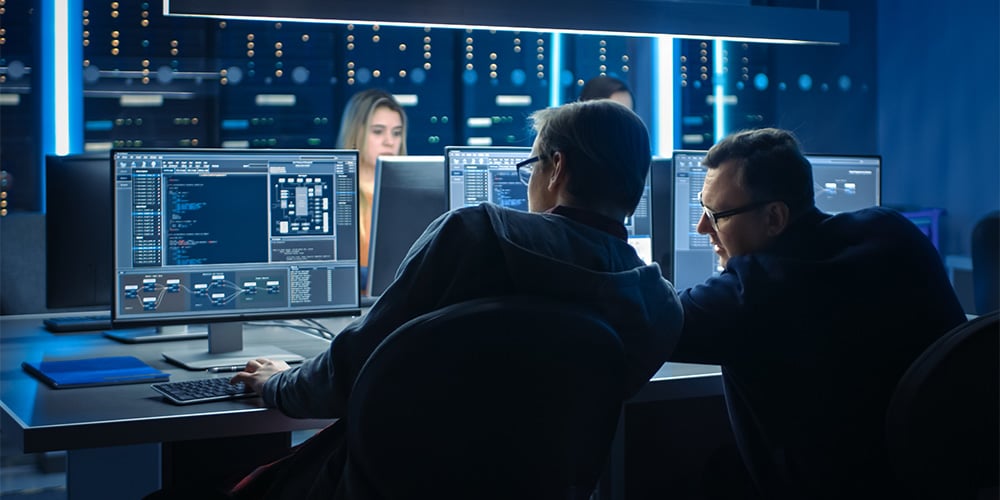
AI and its impact on raising cybersecurity risks
This is the first article in our series on cybersecurity risks in AI adoption based on the whitepaper developed by our colleagues in Grant Thornton US which you can download in full.
Artificial Intelligence (AI) needs no introduction, having managed to rapidly creep into all aspects of life. While in business, AI is creating a plethora of new potential opportunities and efficiencies, it is also presenting new challenges, including in the area of cybersecurity. So how exactly has AI impacted cybersecurity and what are the key risks that can be identified in its present form?
Download Grant Thornton's whitepaper 'Control AI cybersecurity risks'
As AI tools become more prevalent and sophisticated, they can be used to pose significant cybersecurity risks. Threats, such as vulnerability exploitation, malicious software, collusion, extortion and phishing are heightened as AI can be used to evade detection. Traditional security approaches and countermeasures are not sufficient to protect against new and increased risks, such as:
- Incorrect or biased outputs,
- Vulnerabilities in AI-generated code,
- Copyright or licensing violations,
- Reputation or brand impact,
- Outsourcing or losing human oversight for decisions, and
- Compliance violations.
Implementing AI technologies in your organisation can introduce five primary cybersecurity risks. This article sets out the five key risks and their mitigating factors:
1. Data breaches and misuse
Data breaches pose a significant cybersecurity risk for AI platforms that store and process vast amounts of confidential or sensitive data such as personal data, financial data and health records.
Several risk factors can contribute to data breaches in AI platforms. Internally, AI instances that process and analyse data can be vulnerable due to weak security protocols, insufficient encryption, inadequate monitoring, external telemetry, and insufficient access controls. Externally, AI solutions and platforms can be vulnerable to various security risks and can be targets for data theft, especially if the data used to interact with these platforms is recorded or stored.
The risk of misuse and data loss has increased due to the unfettered availability of Generative AI (GenAI). The risk is especially high for IT, engineering, development and even security staff, who may want to use GenAI to expedite their daily tasks or to experiment with new technology. They can inadvertently feed sensitive data through browser extensions, APIs or directly to the GenAI platform. Without an enterprise-sanctioned solution and an acceptable use policy, there is potential that employees could cause an accidental data breach, and commit to terms of use that do not comply with company policy and data protection regulatory requirements.
2. Adversarial attacks
Adversarial attacks manipulate input data to cause errors or misclassification, bypassing security measures and controlling the decision-making process of AI systems. There are several forms of adversarial attacks, and two of the most common types are evasion attacks and model extraction attacks.
Evasion attacks try to design inputs that evade detection by the AI system's defences and allow attackers to achieve their goals (e.g., bypassing security measures or generating false results). Since the inputs appear to be legitimate to the AI system, these attacks can produce incorrect or unexpected outputs without triggering any detection or alerts. Model extraction attacks attempt to steal a trained AI model from an organisation and use it for malicious purposes.
The impact of adversarial attacks vary by use case and industry, but they can include:
- Errors or misclassifications in the output for medical diagnostics, where adversarial attacks can misdiagnose cases and potentially cause improper treatment. In the context of automated vehicles, such attacks could include lane elimination attacks or fake lane attack. These involve manipulation of road markings so that automated cars incorrectly interpret the markings, causing road accidents when using autopilot. Similar attacks can be carried out on traffic signs.
- Decision-making manipulations that could coerce a system into divulging sensitive information or performing unauthorised actions
3. Malware and ransomware
Malware and ransomware have been amongst the greatest threats to IT systems for many years, and the use of AI can make the risks even more prevalent. AI can be used to generate new variants of malware at a quicker rate, for lower costs and with less required expertise by the attacker. The risks for any solution include:
- Disruption of services, caused by encrypting data or overloading networks to prevent legitimate access.
- Hijacking resources for crypto mining or a botnet attack.
- Exploiting publicly available AI platforms to gain access to your network and cause harm.
4. Vulnerabilities in AI infrastructure
As with any software, AI solutions rely on components of software, hardware and networking - all of which can be subject to attack. In addition to traditional attack vectors, AI can be targeted through cloud-based AI services, Graphic Processing Units (GPUs) and Tensor Processing Units (TPUs).
GPUs and TPUs contain specialised processors that accelerate AI workloads, and they can introduce new attack vectors. Design flaws in processors and other hardware can affect a range of products.
AI solutions are also built upon and integrated with other components that can fall victim to more traditional attacks. Compromises to the software stack can trigger denial of service, allow unauthorised access to sensitive data or gain access to your internal network.
5. Model poisoning
Adversarial attacks target AI models or systems in their production environment, whereas model poisoning attacks target AI models in the development or testing environments. In model poisoning, attackers introduce malicious data into the training data to influence the output — sometimes creating a significant deviation in behaviour from the AI model.
For example, after a successful model poison attack, an AI model may produce incorrect or biased predictions, leading to inaccurate or unfair decision making. Some organisations are investing in training closed Large Language Model (LLM) AI to solve specific problems with their internal or specialised data. Without appropriate security controls and measures in place, these applications are vulnerable to model poisoning attacks. In addition to this, many LLMs are not compliant with existing data privacy regulations and therefore there is an inherent risk for organisations that leverage them.
Model poisoning attacks can be challenging to detect, because the poisoned data can be innocuous to the human eye. There are additional detection complexities introduced for AI solutions that leverage open-source or external components, which the majority of solutions today do.
Download Grant Thornton's whitepaper 'Control AI cybersecurity risks'
How Grant Thornton can help
As companies adapt their business strategies for new AI capabilities, they must also adapt their risk management strategies. Cybersecurity, data privacy, and effective controls are critical to the successful business use of AI.
At Grant Thornton, our cybersecurity team have extensive experience in advising organisations on a wide range of risk and technology matters, keeping organisations at the forefront in managing their risks from current and emerging technology.
For more information on our service offering, get in touch with our cybersecurity leaders.
Get the latest insights, events and guidance for Cybersecurity, straight to your inbox.